Client Background
A leading process engineering company provides design, applied chemical engineering, and safety services to a wide range of process industries across the world. These include petroleum refining, chemical production, alternative fuels, food grade products, nuclear materials processing, and many more.
One of their client wants to transition from reactive to proactive maintenance, for smoother operations and productivity.
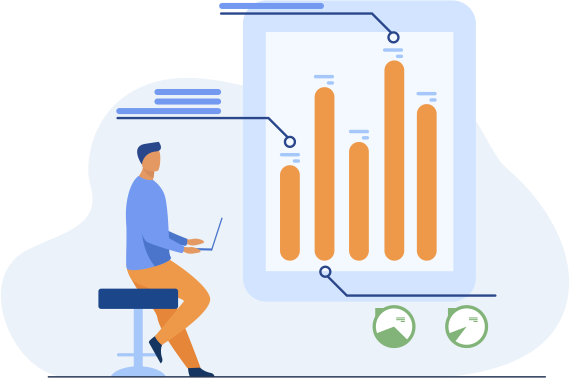
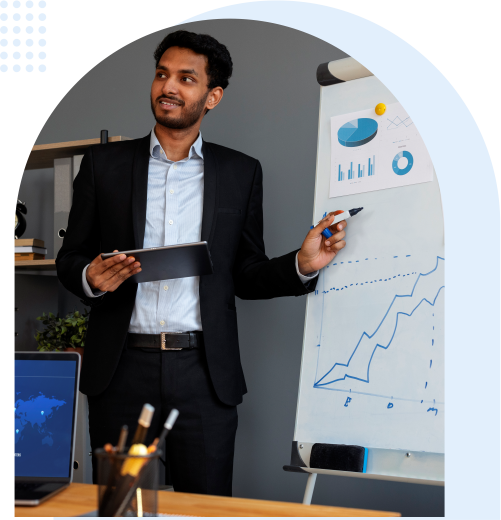
Challenges
- Frequent equipment failures leading to unplanned downtime.
- High maintenance costs due to reactive repair strategies.
- Lack of real-time insights to predict and prevent issues
- Inefficiencies in production processes affecting overall productivity.
- Difficulty in optimizing maintenance schedules and resource allocation.
Strategic Approach
We implemented a artificial intelligence based predictive analytics solution using machine learning algorithms to monitor and analyze equipment performance data.
This system continuously collected data from various sensors installed on critical machinery. By applying predictive models, the system could forecast potential equipment failures, identify inefficiencies, and recommend preventive actions.
Impact
- Reduction Downtime 20% reduction in unplanned equipment breakdown
- Enhanced 10% process efficiency
- Cost Savings Optimized maintenance schedule lowered cost by 15%
- Real Time Monitoring Helps in proactive issue resolution
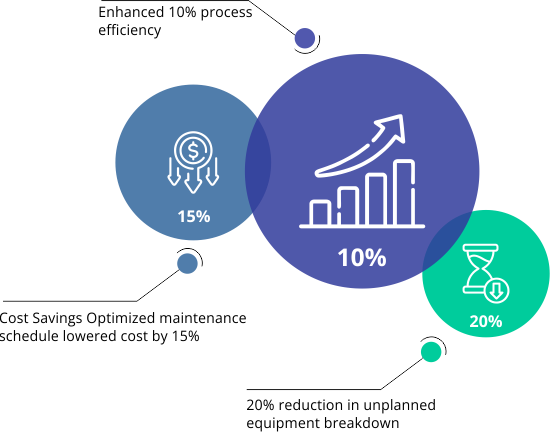
Boost your operations with AI-driven proactive maintenance.